In today’s fast-paced world, companies receive vast amounts of data every minute: clicks, views, transactions, interactions, and more. However, most businesses lack the resources or time to process all this information manually. That’s where Artificial Intelligence (AI) comes in.
According to Exploding Topics, 48% of businesses already use some form of AI to manage big volumes of data effectively. Moreover, when combined with Business Intelligence (BI), AI fundamentally enhances companies’ data analysis and decision-making processes.
At Devtorium, we have outstanding Data Science and Business Analytics experts who specialize in enhancing companies’ data processing. With extensive experience in providing AI-driven BI tools, our leading specialist, Olena Medvedieva – Head of the Data Science Department, has prepared a blog on the efficient application of AI in BI.
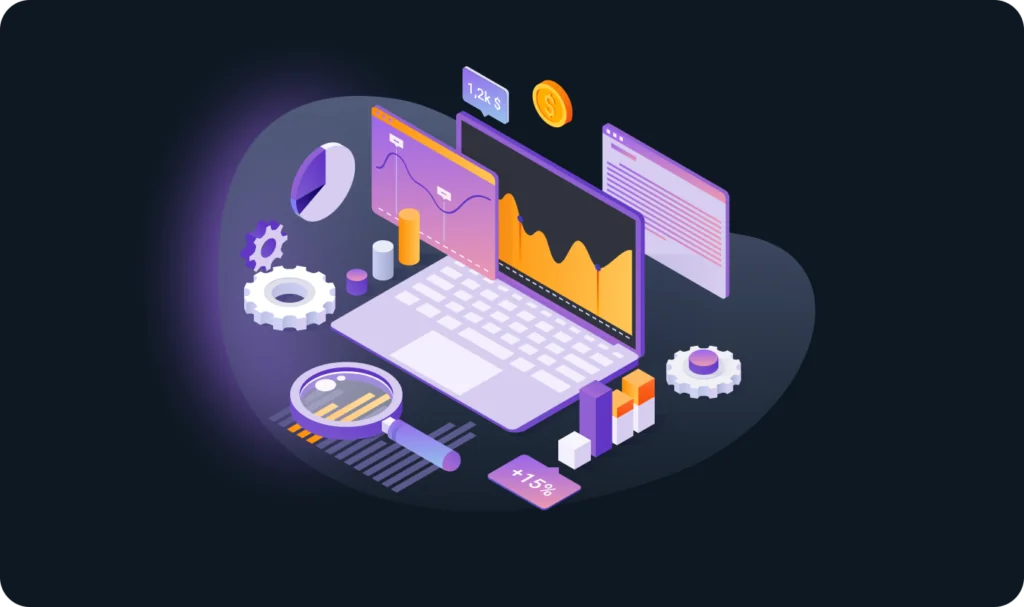
AI Modernizing BI Tools
At its core, Business Intelligence is about transforming raw data into valuable insights that help businesses make better decisions. BI tools help companies track performance, monitor key metrics, and analyze past data to understand trends. But while BI has been great at showing what happened, AI is now stepping in to show why and what might happen next. So, how exactly does AI fit into the BI picture?
1. Smarter Data Analysis
Traditional BI tools focus on structured data, such as sales numbers or customer demographics. However, AI can analyze all types of data, including unstructured ones like emails, social media posts, or audio recordings. By examining a fuller picture of data, businesses can uncover hidden insights and make more informed decisions.
2. Predicting the Future
AI isn’t just good at analyzing what happened; it’s great at predicting what will happen next. Using machine learning, AI can examine past data to forecast trends, customer behavior, and potential risks. For example, a retailer could predict which products will be in high demand next month, helping avoid stock shortages or overstocking – ultimately saving time and money.
3. Natural Language Processing (NLP)
One of the most impressive aspects of AI in BI is its ability to interact with data in a more natural way. With Natural Language Processing (NLP), you can ask your BI system questions in plain language, just like you would ask a colleague. For example, you could ask, “What were the top-selling products last week?” and get a quick, clear response—no need to be an expert in data or learn complicated commands.
4. Automating Insights
AI can also identify and highlight essential insights. By continuously scanning data for trends or anomalies, it can alert you to unusual occurrences, such as a sudden drop in sales or a spike in customer complaints. These insights help businesses stay on top of critical real-time changes and react faster.
5. Improved Data Visualization
By recommending the best way to visualize data, AI makes it easier for businesses to see trends and patterns. Whether through bar charts, line graphs, or heat maps, AI ensures that presented data is in the most insightful and accessible way possible.
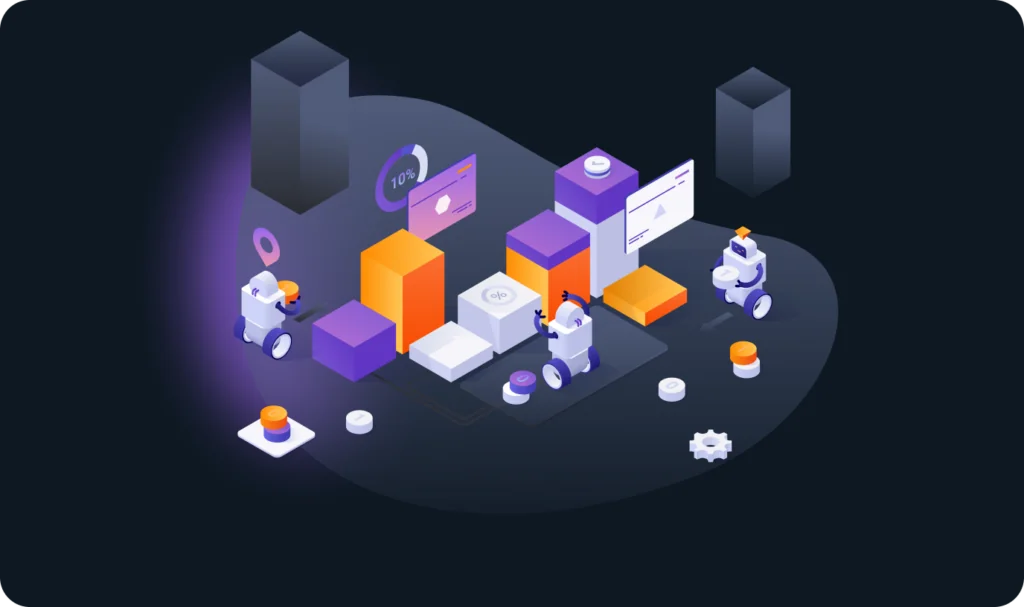
The Strategic Advantages of AI in BI
Adding AI into the BI area drives businesses to an entirely new level. By doing so, companies benefit from various fields, empowering them to stay ahead in today’s data-reliant market. Here’s how:
- Faster, Smarter Decisions by processing enormous amounts of data quicker and more accurately than doing that manually.
- Cost Savings by automating tasks that traditionally require manual effort, such as data analysis and report generation. AI can free up employees to focus on more strategic tasks, ultimately saving money and boosting efficiency.
- Scalability by scaling solutions as the business grows. It helps companies handle more extensive datasets and complex analyses, ensuring that data-driven decision-making remains possible even as the business expands.
Challenges to Consider
Of course, integrating AI with BI isn’t all smooth sailing. There are a few challenges businesses need to consider:
- Data Quality: AI is only as good as its fed data. If the data is inaccurate, incomplete, or biased, the insights AI provides may not be reliable. Businesses need to ensure that their data is clean and high-quality.
- Cost and Expertise: While AI-powered BI tools are becoming more accessible, they still require a significant investment. Not to mention, finding professionals who can integrate and manage these tools requires specialized skills.
- Ethics and Privacy: As with any technology, AI raises ethical concerns, especially regarding data privacy. Businesses must be transparent about collecting, storing, and using data to avoid privacy violations or bias in their AI models.
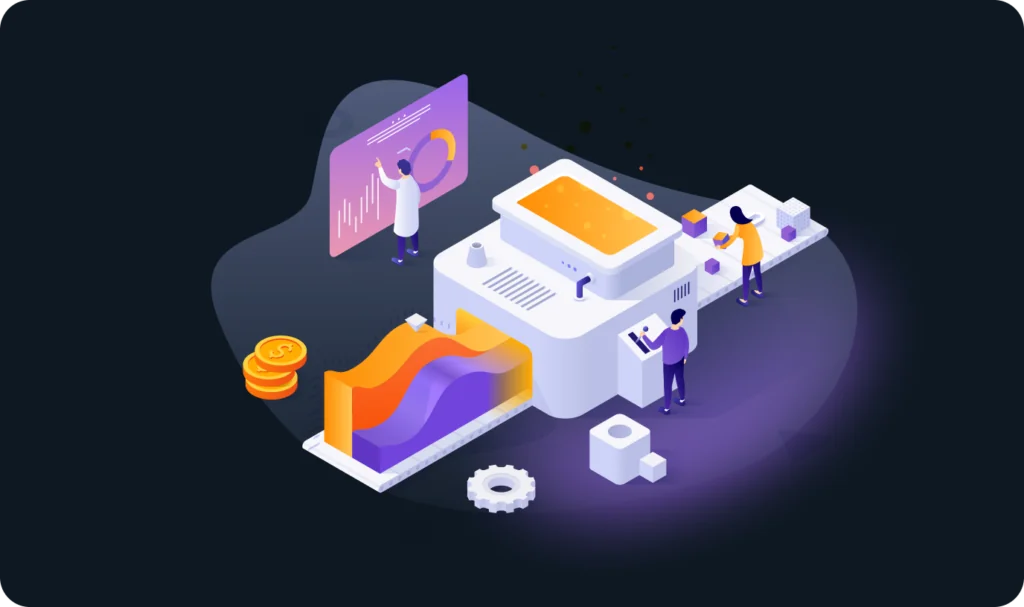
Bottom line: Why AI is the Perfect Fit for Business Intelligence?
Overthinking the future of AI in BI, it is predictable how manageable and robust working with data will become. Moreover, their combination will help businesses quickly uncover trends, predict future outcomes, and automate repetitive tasks, freeing time for more strategic thinking. With user-friendly AI tools, even those without technical backgrounds can gain valuable insights from data.
To see how AI and BI tools transform your business in practice, book a free consultation with the Devtorium Data Science team!
More on AI from Devtorium: